Background
The current study explores digital online learning in the context of the effects Blacks and Latinos face during the COVID-19 pandemic. The World Health Organization’s pronouncement of the COVID-19 as a global issue resulted in the suspension of physical learning, which, in turn, paved the way for remote learning via the Internet (Lwin et al., 2020). Even though online learning was essential, especially in promoting education, it revealed differences among learners from various communities. This disparity in the Internet access is revealed by the data obtained from the United States Census Bureau 2021 survey on computer and Internet availability in households with children in public or private school, by select characteristics. The data is useful for this topic as it can help policymakers and service providers enable equality to the Internet access.
The data obtained from the United States Census Bureau 2021 survey (see Appendix) provides insight on various issues regarding the Internet access. For instance, it informs on the knowledge regarding the access to computer and the Internet. It also explores different parameters such as age, gender, race, level of education, marital status, presence of children, computer and internet providers, employment status, and household income. This data points are crucial in understanding how different categories of people access online learning resources, thus, making possible interventions to reduce the disparity between the various learners. This study expects to find that the Blacks and Latinos have lower internet access compared to other races. It is also critical to note that the one who has internet access may not necessarily have a computer, though having one makes the Internet consumption more productive in learning.
Critical Question Answers
This section explores various areas of the survey, including reliability, validity, independent and dependent variables, outliers, and answers whether the research expectation is achieved.
Reliability
Reliability of data refers to the completeness and accuracy of information collected from the field. It indicates that the gathered data meets the purpose for which it is intended, and that it is not subjected to various kinds of alterations to fit any specific purpose. According to Mohajan (2017), reliability can be achieved by conducting several tests or surveys and when the collected data consistently reveal the same outcome, then one can infer the data to be reliable. Reliability analysis is done by finding a consistent variation in proportion in a given scale, which can help in establishing the association between various scores found from different tests within that scale (Barrows et al., 2019). Consequently, a high association in the analysis scale gives a consistent result, which reveals the reliability of the data.
To measure the reliability of the data used in the current survey (see Appendix), this study uses the Cronbach’s alpha. According to McNeish (2018), Cronbach’s alpha measures the internal consistency of several data points. Viladrich et al. (2017) reveal that various values of alpha indicate the level of data validity, hence the choice to consider the dataset valid or not. Therefore, validity shows how closely various data points of a dataset relate. Specifically, Microsoft Excel is used to perform this analysis, since it provides codes and internal functionalities for this task. The Cronbach’s alpha, is given by the following formula:
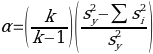
Where:
- k is the number of tests conducted,
is the variance of the scores, and
is the sum of the individual variance.
- K= 6
= 1.68082E+14
= 1.10709E+14 for computer availability and
= 1.03077E+14 for internet availability
Therefore,
= 0.409609938.
On the other hand, the internet availability’s coefficient is given by
= 0.464092965
The values obtained for the reliability of the data on the Internet and computer accessibility indicate that the data is unreliable. However, this happens in cases where various respondents did not provide answers for some survey questions. This was evident in all the questions asked, which affected the data’s reliability.
Validity
Validity of a dataset refers the extent to which its truthfulness can be determined. Thus, the validity of a survey asserts or measures the specific theory claimed in the study. According to Streiner (2020), the validity of any dataset can be categorized as either internal or external. Internal validity mainly describes the degree to which the observed parameters are certain in any specific research and are the actual results of the survey. Thus, it removes any chances of incorporating possible interventions, which can affect the outcome (Pound & Ritskes-Hoitinga, 2018). Therefore, it can be improved by increasing control of different variables used in the study. On the other hand, external validity mainly describe the extent to which the outcomes of a research are applicable in real world, where it is beyond the control of the study (Wang et al., 2020). Hopkins (2017) indicates that the validity of a dataset can be measured using correlation. However, due to the nature of the dataset used in the current study, the validity is measured only on the racial aspects regarding access to the Internet and computer. The following tables 1 and 2 give the correlation outputs based on the Microsoft excel output.
Independent and Dependent Variables
Every experiment has two different variables, which include dependent and independent variable. A dependent variable is the one, which is under investigation in any specific study. On the other hand, an independent variable is one the, which is controlled in the survey to affect the dependent variable (Bloomfield & Fisher, 2019). Thus, changing values of the independent variable have consequences on the dependent variable. For example, in the current survey data, the independent variable is race while the dependent variables are the access to computer and the Internet. Changing racial backgrounds affects accessibility of computer and the Internet since it results in different outcomes in various races.
Outliers
Any survey conducted usually have data ranging from the lowest to the highest values. An outlier refers to the observations, which occupy abnormal distances from the rest of the data collected in the sample. To identify such values in a survey data, parameters such as median, lower and upper quartiles, interquartile range, lower and upper inner fences, and lower and upper outer fences are analyzed. Based on the dataset used in this analysis, the following values given in Table 3 were obtained.
The analysis above indicates that the values 373 and 39,611,510 are the outliers as they are the way below and above other data points, respectively. Thus, this study reveals that the Latinos and Blacks have lower access to internet, hence face more issues regarding online learning.
References
Barrows, C., Bloom, A., Ehlen, A., Ikäheimo, J., Jorgenson, J., Krishnamurthy, D., Lau, J., McBennett, B., O’Connell, M., Preston, E. & Staid, A. (2019). The IEEE reliability test system: A proposed 2019 update. IEEE Transactions on Power Systems, 35(1), 119-127. Web.
Bloomfield, J., & Fisher, M. J. (2019). Quantitative research design. Journal of the Australasian Rehabilitation Nurses Association, 22(2), 27-30. Web.
Hopkins, W. G. (2017). Spreadsheets for analysis of validity and reliability. Sportscience, 21(9), 36-44.
Lwin, M. O., Lu, J., Sheldenkar, A., Schulz, P. J., Shin, W., Gupta, R., & Yang, Y. (2020). Global sentiments surrounding the COVID-19 pandemic on Twitter: Analysis of Twitter trends. JMIR Public Health and Surveillance, 6(2), e19447.
McNeish, D. (2018). Thanks coefficient alpha, we’ll take it from here. Psychological Methods, 23(3), 412. Web.
Mohajan, H. K. (2017). Two criteria for good measurements in research: Validity and reliability. Annals of Spiru Haret University. Economic Series, 17(4), 59-82. Web.
Pound, P., & Ritskes-Hoitinga, M. (2018). Is it possible to overcome issues of external validity in preclinical animal research? Why most animal models are bound to fail. Journal of Translational Medicine, 16(1), 1-8. Web.
Streiner, D. L. (2020). Statistics commentary series. Commentary no. 44: Internal and external validity. Journal of Clinical Psychopharmacology, 40(6), 531-533.
Viladrich, C., Angulo-Brunet, A., & Doval, E. (2017). A journey around alpha and omega to estimate internal consistency reliability. Annals of Psychology, 33(3), 755-782.
Wang, L., Graubard, B. I., Katki, H. A., & Li, A. Y. (2020). Improving external validity of epidemiologic cohort analyses: a kernel weighting approach. Journal of the Royal Statistical Society: Series A (Statistics in Society), 183(3), 1293-1311. Web.