Rationale
Academic success among students in higher education is motivated by various factors. In the modern world, various techniques and tools are available to researchers to assess the impact of one aspect or another on student success in learning. It is also important that, based on the analysis of various factors, it is possible to predict which of them are most important for academic achievement. Alyahyan and DĂĽĹźtegör (2020) cite that academic success can be defined as “academic achievement, satisfaction, acquisition of skills and competencies, persistence, attainment of learning objectives, and career success” (p. 3). They also highlight the main groups of factors that potentially influence the achievement of academic success. Among them are psychological aspects, demographics, e-learning options, previous academic experience, as well as the environment (Alyahyan & DĂĽĹźtegör, 2020). Analysing these aspects’ impact and interactions can help educational professionals determine the predictors of student success or failure.
In turn, this activity is necessary for the institution to form responses, prevent student academic failures, and ensure better achievements. Rosito (2020) also notes that for students, the ability to control the influence of external factors on their performance leads to greater academic achievements. In this case, if necessary, the prediction of academic success and the transformation of influencers have positive effects on both students and educators (Cachia et al., 2018; Boumi & Vela, 2022). This study seeks to find correlations between various factors potentially influencing students’ academic performance and achievement. The results of this research can later be used to form a framework within which educational institutions can model the most effective educational processes. It is also important that the study will also allow students to pay attention to the factors of influence and independently negate their negative effects or adapt to them.
The purpose of this study was to look at socio-demographic variables (such as gender, age, income, family composition etc.) and student’s recent grades and try to establish using correlation and multiple regression if there is a relationship if this relationship is uni- or multi-dimensional or if no effect can be observed at a level of certainty.
Measures
This study of 97 participants used a combination of single-choice questions, numerical questions and Likert-scale questions which measured agreement against given statements. The online survey used previously validated questionnaires: Learning Self-Regulation Questionnaire (Black & Deci, 2000): respondents were asked to rate on scale from 1 Not at all true to 7 Very true 12 statements related to their reasons for participating actively in their class; General Self-Efficacy Scale (Chen, G., Gully, S. M., & Eden, D. (2001): asking respondents to rate on a scale from 1 Strongly disagree to 5 Strongly agree 8 statements on self-efficacy; Levenson IPC Scale (internality, powerful others, chance; 1974): respondents were asked to rate 24 attitude statements on a scale from minus 3 Strongly disagree to plus 3 Strongly agree; and finally respondents were served a personality test (Goldberg, Lewis R. (1992) The development of markers for the Big-Five factor structure) where they had to rate on a scale from 1 Disagree to 5 Agree, 10 statements about their personality.
The data was coded back for each section: Self-Regulation, the two subscale scores were calculated by averaging the items on that subscale: autonomous regulation (auto.reg): 1, 4, 8, 9, 10 and controlled regulation (cont.reg): 2, 3, 5, 6, 7, 11, 12; Self-efficacy (self.eff) – averaging the responses to the 8 items; Locus of control – consisting of internal (loc.in), power of others (loc.pow) and random (loc.ran), scoring: I = sum of 1, 4, 5, 9, 18, 19, 21, 23; P = sum of 3, 8, 11, 13, 15, 17, 20, 22; C = sum of 2, 6, 7, 10, 12, 14, 16, 24 and Consciousness (con.total) scoring: 10 items 1, 3, 5, 7, 9, 10, and reverse scored 2, 4, 6, 8.
Results
For the purposes of the study, the following variables were analysed: for multiple regression Recent Grades (dependent variable) and edu.level, age, no.depend, easy.time, support closest, gender (independent variables); for correlation, the following variables were correlated: auto.reg, cont.reg, self.eff, loc.int, loc.pow, loc.ran, con.total and recent.grades.
The sample of the study consisted of 97 students (78% females, 18% males and 4% others) of ages 18 and over (21% aged 18-25, 25% aged 26-30, 30% aged 31-35, 12% aged 36-40 and 12% aged 41 or over), all students.
Descriptive analysis was performed on all 97 respondents to identify outliers and incomplete, and whilst cases of incomplete answers (4) and possibly outliers (3) were flagged, they were not enough to affect the reliability of our data analysis, as such the regression and correlation was ran on the full data of 97 respondents. The recent grades exploratory analysis showed a mean M=5.24 and a standard deviation SD=1.61, with a good normal distribution.
The reliability analysis, Cronbach’s alpha was run for each scale, and it determined that the scales used were good or good and acceptable, but we have not got a Cronbach’s alpha over 0.7 in any scale. Cronbach’s alpha if deleted was also suggestive that the scales wouldn’t not improve if items deleted, pointing again that scales could not be improved by omitting items; we present below the Cronbach’s alpha for self-regulation scales, where no above 0.7 score was achieved.
Next, we move on to perform the correlation analysis on auto.reg, cont.reg, self.eff, loc.int, loc.pow, loc.ran, con.total and recent.grades. The SPSS results showed:
* = significant at 0.05; ** = significant at 0.01; *** = significant at 0.005
No direct correlation between recent grades and any other of the variables included was established; however, we have observed negative correlations between controlled regulation and self-efficacy (significant at 0.01) and a positive with locus of control loc.pow (significant at 0.05). In fact loc.pow was the strongest correlated variable, achieving significant correlations with cont.reg (significant at 0.01), self.eff (significant at 0.05), loc.in (significant at 0.05) and loc.ran (significant at 0.05).
Finally, we have also run multiple regression analysis between Recent Grades (dependent variable) and edu.level, age, no.depend, easy.time, support closest, gender (independent variables):
Analysing the output from the table – we can observe that edu.level, age and support.closest are significant predictors of recent.grades, with sig. p-values below.05 (p <0.5). In terms of how strong the effects are, for a 1-unit change in age, we expect a -0.34 change in recent.grades, and for a 1-unit change in edu.level, we expect a 0.23 change in recent.grades. Collinearity wise all values are less than 10 – indicating no issues with collinearity. In fact only one condition threw a value of 15, pointing towards a possible issue:
Discussion
The study’s data analysis has provided us with an insight in how social variables in an individual’s life can influence its academic achievement and performance. It uncovered that performance in education can be mostly affected by demographic factors such as education level, and in some small measure, support from the close ones and age.
Whilst self-regulation and self-efficacy can also play a role, the study could not find a direct correlation between these variables and educational performance, it however uncovered that self-regulation and locus of control (internality, power of others, random) can correlate with each other. But is this a force that can predict the direction of how a student would perform in exams? The study cannot prove this theory, and it only offers the potential for further inquiry into this aspect.
Whilst it is easy to predict that age, level of education, support of others would affect how a student performs, it’s not simple to determine a relation when the factors involved are more intrinsic and themselves dependent on other factors. Baba et al. (2018) notes that there is a correlation between factors such as gender and age and students’ academic performance. While age appears to have little effect on academic success, gender appears to be a significant correlate. At the same time, the authors note that, taken together, these factors act as an ambiguous predicate of academic achievement, which does not allow them to be used as reliable data. For example, for older males, the correlation is much higher than for younger females, which identifies the mutual influence of demographic aspects on each other. Thus, relationships between factors in predicting academic success are likely to be more important than individual characteristics.
An association of gender and an increased risk of lower academic success has also been identified by other researchers. Nawa et al. (2020) underline that “males were significantly associated with being in a group of students who withdrew or repeated years” (p. 7). Additionally, the authors found a correlation between educational attainment and student achievement in high school and academic achievement in higher education (Nawa et al., 2020). This study establishes correlations between individual characteristics and academic success, but also does not consider the interaction of indicators. Thus, this aspect is the least studied at the present time and is promising for future research.
Further contributing to the weak findings of this study, are an array of factors such as sample composition (only respondents with online access could take part, a short period of time was offered to take part), survey design (scales in the questions offered were not consistent, some ranging from 1-5, others from -3 to 3 and some from 1 to 8, could confuse respondents to measure themselves uniformly) and potentially a lack of direction (the study tries to establish that educational achievement is influenced by socio-demographic factors, but fails to establish if the way educational achievement is measured is a correct and representative system).
The Cronbach’s alpha low score could potentially be a safe predictor of the weak results obtained and should allow future researchers that would replicate the survey to improve on its design, sample composition and hypothesis tested. A score of 0.8 or above is needed to be able to make a reliable inferential analysis on the data collected.
Reference
Alyahyan, E., & Düştegör, D. (2020). Predicting academic success in higher education: Literature review and best practices. International Journal of Educational Technology in Higher Education, 17(3), 1-21. Web.
Baba, I., Aliata, M., & Patrick, B. (2018). Demographic factors and students’ academic achievement in tertiary institutions in Ghana: A study of Wa Polytechnic. Journal of Education and Practice, 4(20), pp. 76-80.
Boumi, S., & Vela, A. (2022). Impacts of students academic performance trajectories on final academic success. Computers and Society, 1-8. Web.
Cachia, M., Lynam, S., & Stock, R. (2018). Academic success: Is it just about the grades? Higher Education Pedagogies, 3(2), 434-439. Web.
Nawa, N., Numasawa, M., Nakagawa, M., Sunaga, M., Fujiwara, T., Tanaka, Y., & Kinoshita, A. (2020). Associations between demographic factors and the academic trajectories of medical students in Japan. PLoS ONE, 15(5), 1-12. Web.
Rosito, A. C. (2020). Academic achievement among university students: The role of causal attribution of academic success and failure. Humanitas Indonesian Psychological Journal, 17(1), 23-33. Web.
Appendix
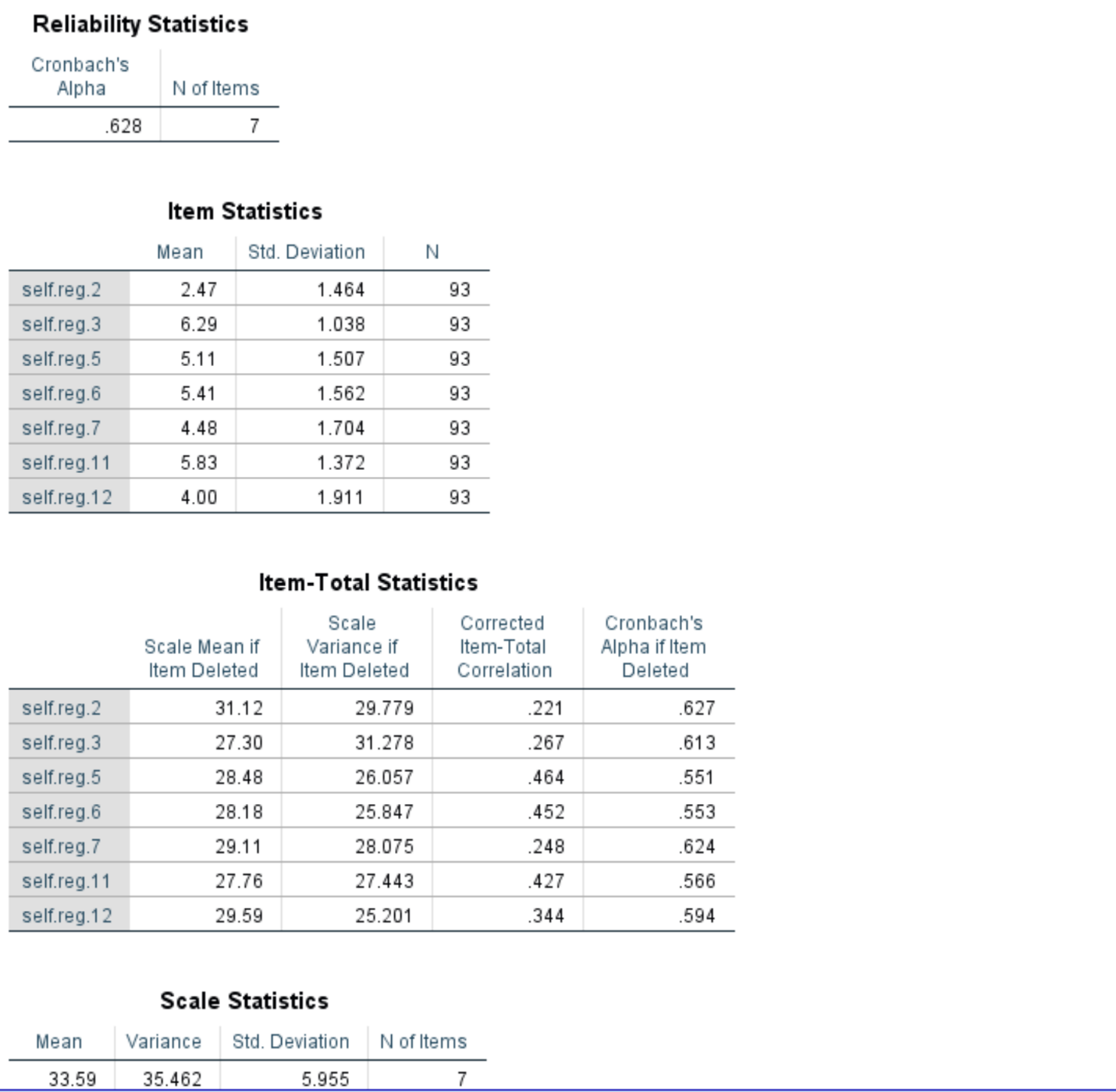
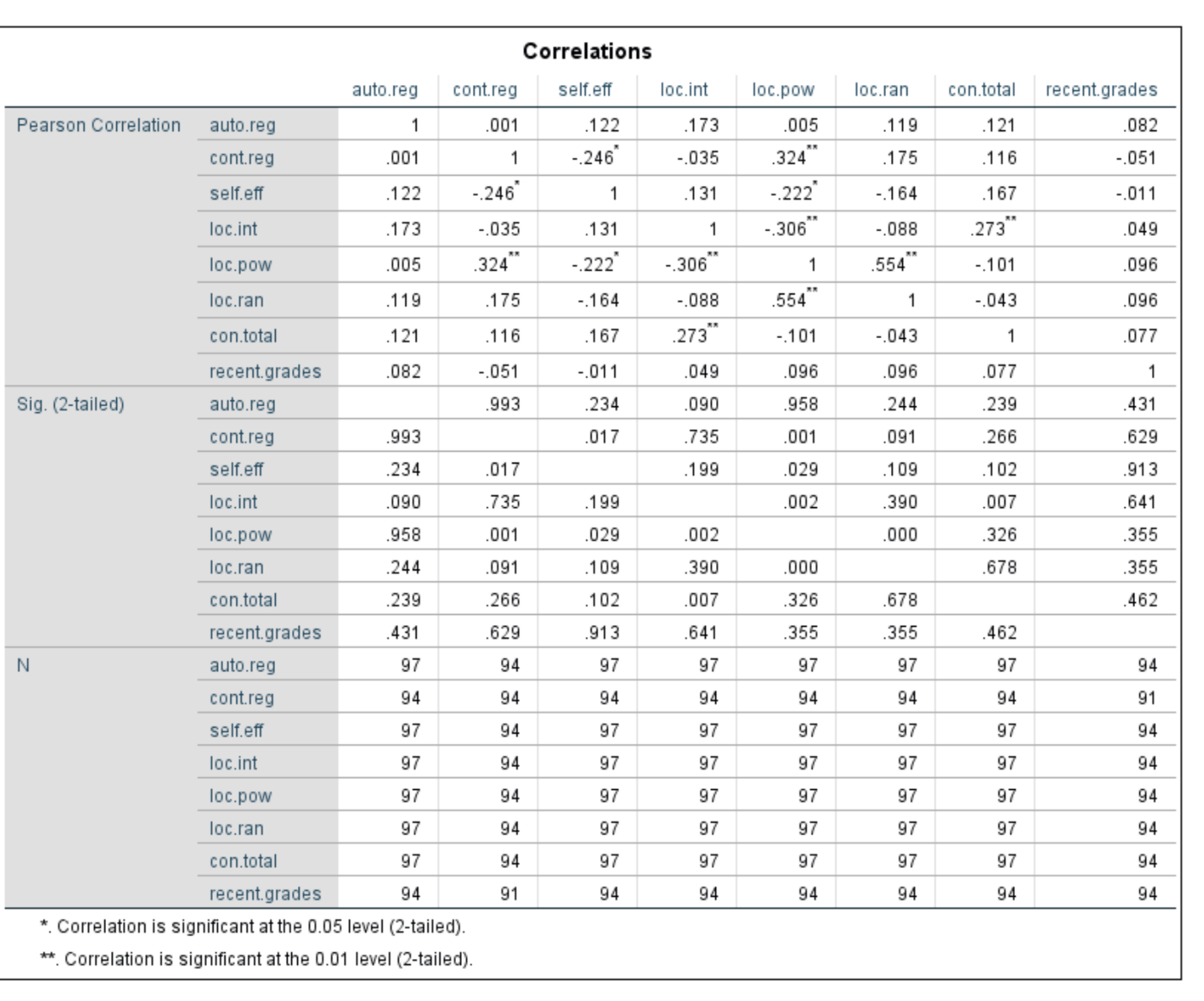
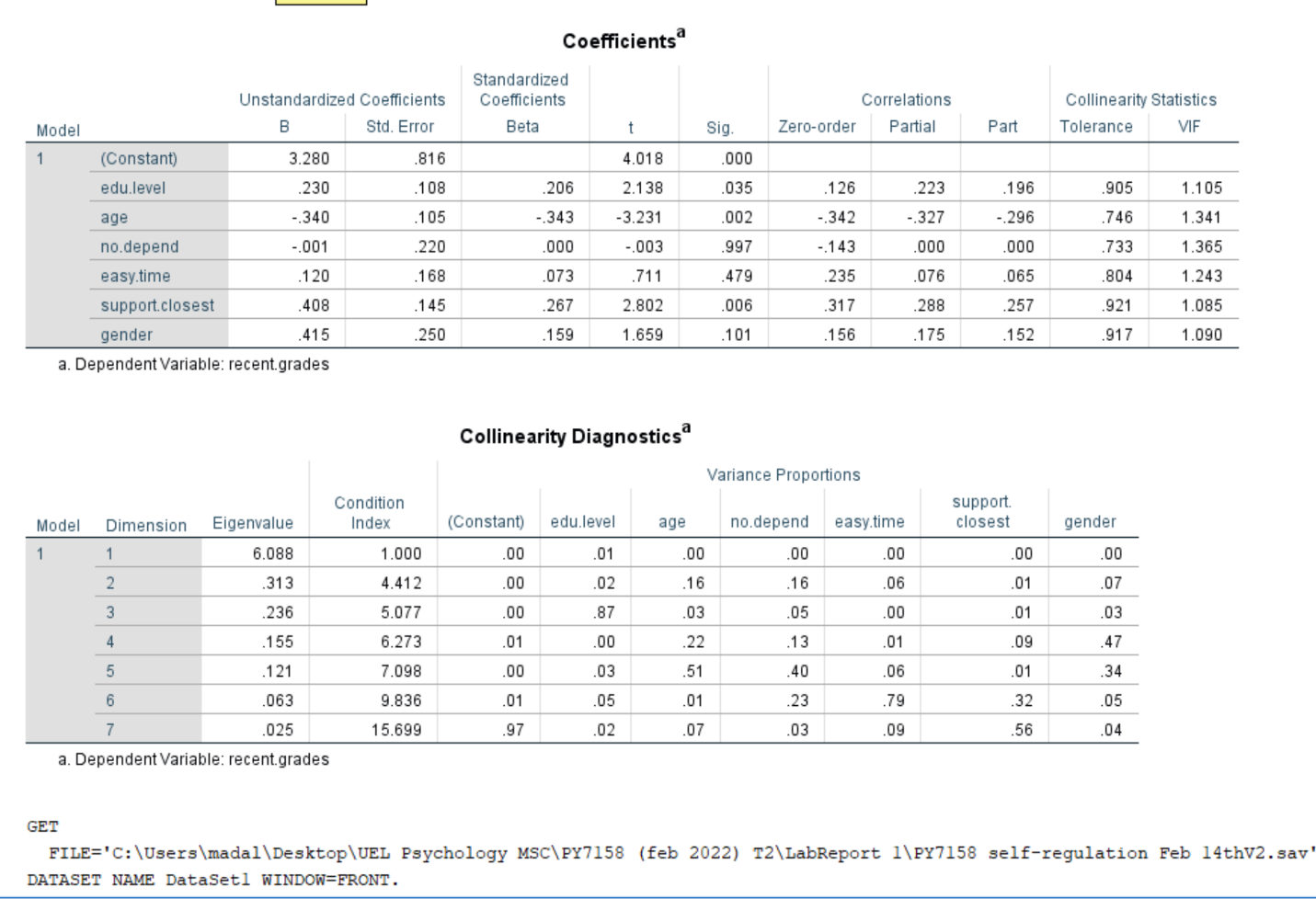